Text Annotation
Named Entity Recognition (NER)
Named Entity Recognition (NER) is a crucial component of natural language processing (NLP) that involves identifying and classifying key entities within text into predefined categories such as names of people, organizations, locations, dates, and more. By leveraging advanced algorithms, NER systems can automatically detect these entities, enabling efficient information extraction and knowledge organization. This capability is essential for applications like search engines, content recommendation systems, and business intelligence, where understanding and categorizing large volumes of text data quickly and accurately is paramount.
Sentiment Analysis
Sentiment Analysis is an AI-driven process used to determine the emotional tone and subjective information expressed in text. By analysing sentiments, businesses can gauge customer opinions, track brand reputation, and gain insights into public sentiment on various topics. This technology helps companies understand customer feedback, improve products and services, and make informed decisions. Sentiment analysis is widely used in customer service, marketing, and social media monitoring to understand and respond to the emotions conveyed in customer interactions.

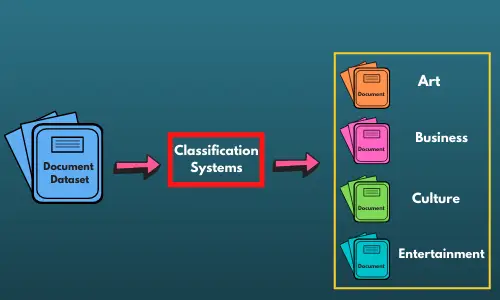
Text Categorization
Text Categorization, also known as text classification, is the process of assigning predefined categories or labels to text based on its content. This technique is vital for organizing large datasets, automating content management, and improving search functionality. Applications include spam detection, news categorization, and document management. By leveraging machine learning models, text categorization systems can efficiently classify vast amounts of text data, ensuring relevant information is easily accessible and actionable.
Intent Recognition
Intent Recognition involves identifying the purpose or goal behind a user’s query or statement. This technology is crucial for creating effective conversational agents, chatbots, and virtual assistants that understand and respond appropriately to user requests. By accurately interpreting user intent, businesses can provide personalized experiences, improve customer service, and streamline interactions. Intent recognition enhances user satisfaction by enabling systems to understand context, anticipate needs, and deliver relevant responses.
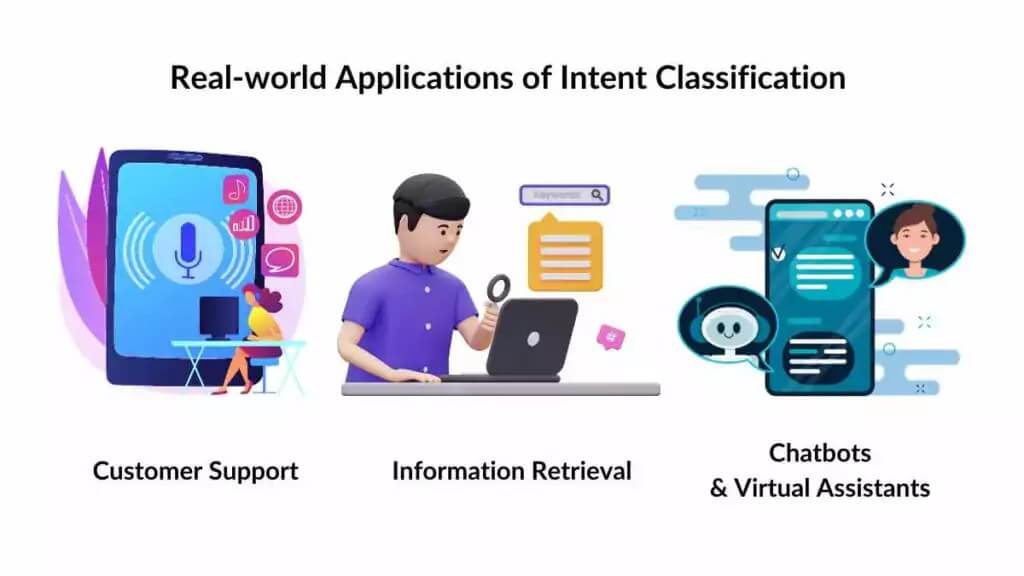

Emotion Analysis
Emotion Analysis, or emotion detection, focuses on identifying and interpreting the emotional states expressed in text. This technology goes beyond sentiment analysis by recognizing specific emotions such as joy, anger, sadness, and surprise. Emotion analysis is valuable in understanding customer experiences, improving human-computer interaction, and enhancing content creation. By detecting emotions, businesses can tailor their communication strategies, develop empathetic AI systems, and create more engaging user experiences. This capability is particularly useful in customer service, mental health applications, and market research to better understand and address user emotions.