You want to succeed, surround yourself with the right kind of people who will support and encourage you all the way. Be with people who have utmost conviction and patience. The battle is never lost until you’ve abandon your vision. But what if you’re really exhausted physically, mentally, and most of all emotionally? Here are some sources of motivation to prompt you in reaching the peak of accomplishment.
Urban life in the city
Never ever think of giving up. Winners never quit and quitters never win. Take all negative words out of your mental dictionary and focus on the solutions with utmost conviction and patience. The battle is never lost until you’ve abandon your vision.
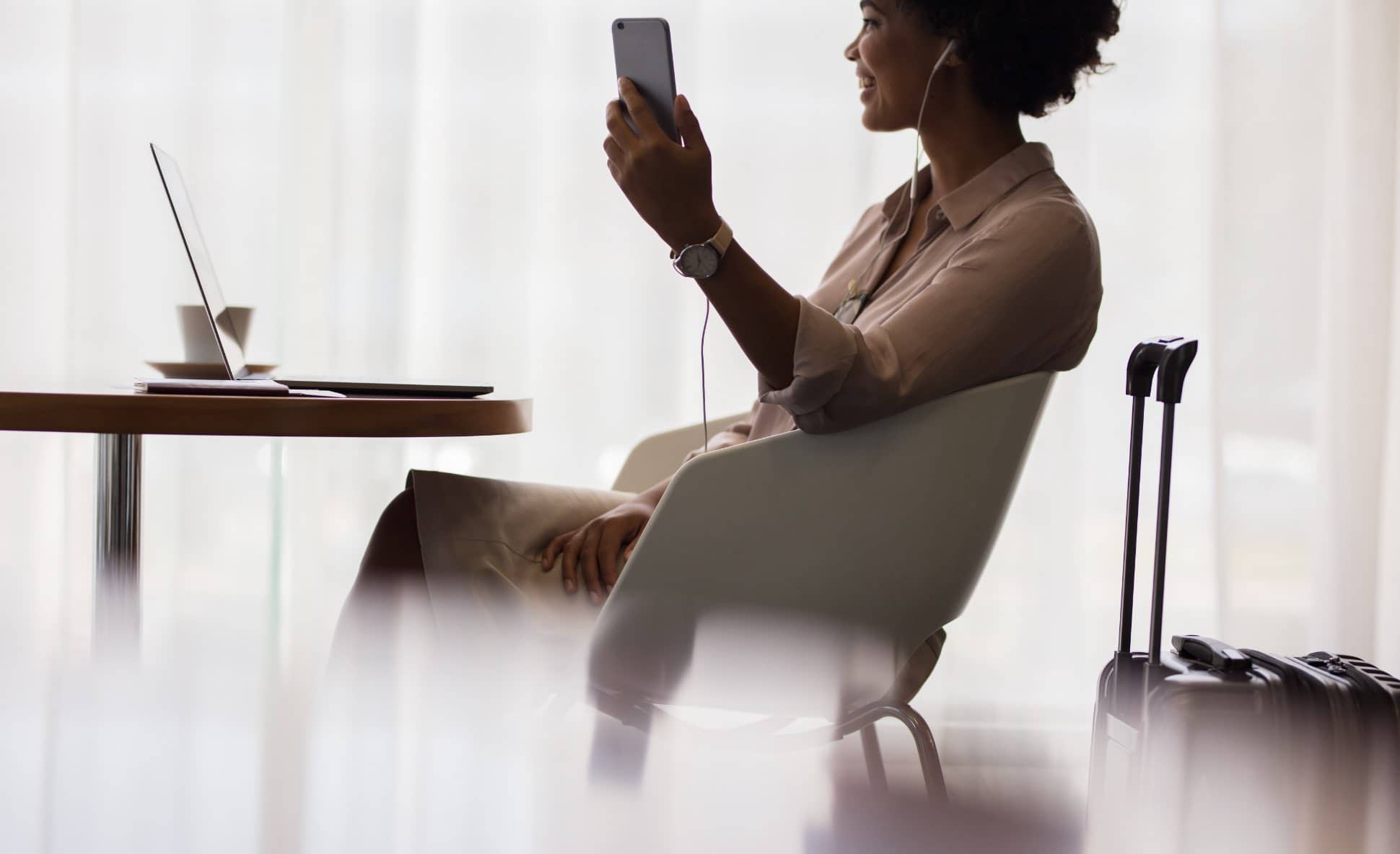
Seeing trends before they start
Success needs hard work. Don’t listen to these ‘get rich quick’ schemes. You need to build your character and work hard on yourself and your business to achieve greatness. Work hard and work smart. Do the right things and do them in the right way. Don’t procrastinate. Take bold actions. Work long hours and craft your legacy.
Learning from failure
Successful people do not see failures as failures. They see them as important learning lessons. Lessons that are capable of giving them insights to prevent such mistakes from happening again. By adopting this mindset of turningExplore the city and new places
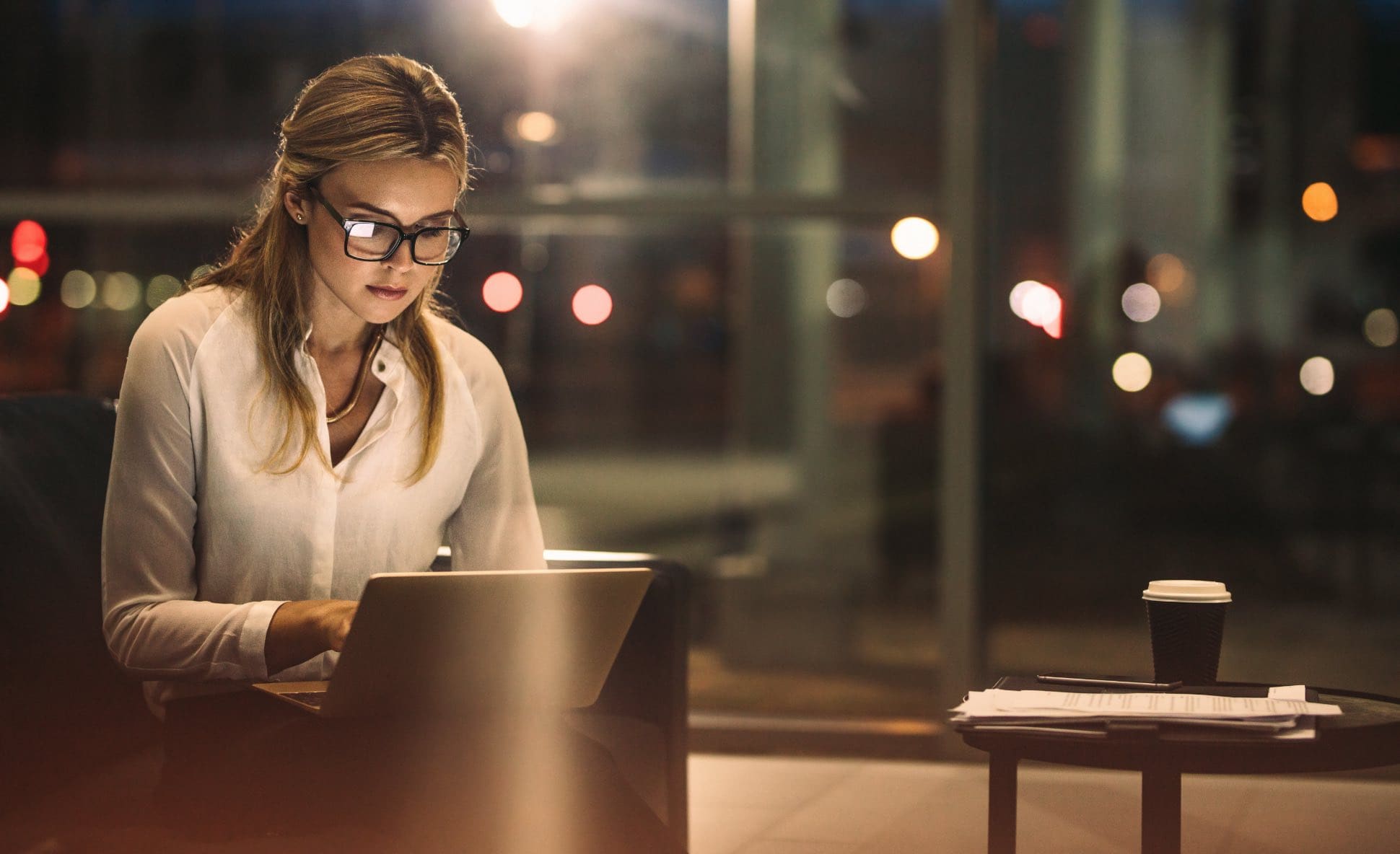
Explore the city and new places
Success needs hard work. Don’t listen to these ‘get rich quick’ schemes. You need to build your character and work hard on yourself and your business to achieve greatness. Work hard and work smart. Do the right things and do them in the right way. Don’t procrastinate. Take bold actions. Work long hours and craft your legacy.
Do something that keeps you live
Successful people do not see failures as failures. They see them as important learning lessons. Lessons that are capable of giving them insights to prevent such mistakes from happening again. By adopting this mindset of turning