Generative AI, a subset of artificial intelligence, has shown incredible promise across various industries, including health care.
By leveraging deep learning models such as Generative Adversarial Networks (GANs) and large language models, generative AI has the potential to reshape how medical professionals approach diagnostics, treatment planning, and even drug discovery.
In this blog, we explore the impact of generative AI in health care, supported by facts and statistics.
What Major Role Does Generative AI in Health Care Play?
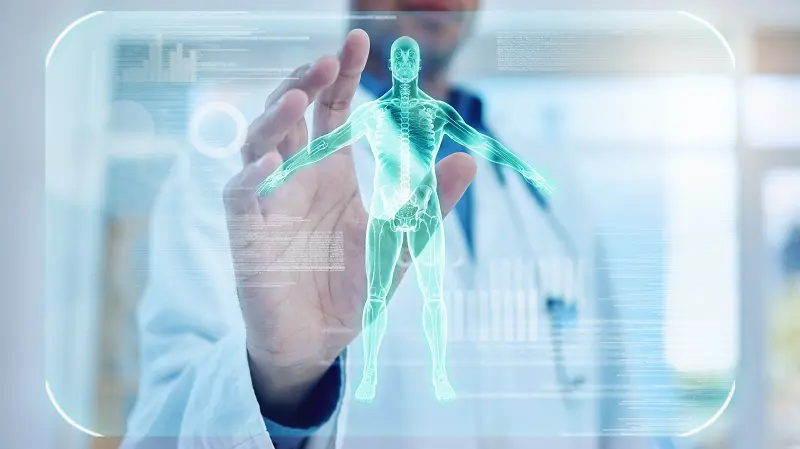
Generative AI involves algorithms capable of creating new data resembling the input data they are trained on. This technology is revolutionizing health care by enabling novel applications in:
1. Medical Imaging and Diagnostics
Generative AI is transforming medical imaging, allowing for the enhancement of image quality, filling gaps in imaging data, and even generating synthetic datasets for training purposes. For example, GANs can create high-resolution images from low-quality scans, aiding radiologists in making more accurate diagnoses.
Statistic: According to a report, the global AI in medical imaging market is projected to reach $20 billion by 2030, growing at a compound annual growth rate (CAGR) of 34.9% from 2021 to 2030. (Source: Grand View Research)
2. Drug Discovery and Development
Generative AI in Healthcare accelerates drug discovery by identifying new molecular structures, predicting drug interactions, and optimizing clinical trial designs. Deep learning models can generate millions of drug-like compounds, significantly reducing the time and cost associated with drug development.
3. Personalized Medicine
Generative AI enhances the creation of tailored treatment plans by simulating patient-specific scenarios. For instance, AI models can predict how individual patients will respond to certain drugs based on genetic and historical data.
4. Virtual Health Assistants
Generative AI powers virtual health assistants that provide patients with real-time support, answer medical queries, and schedule appointments. Large language models like GPT-4 enable these assistants to communicate with patients in a conversational and empathetic manner.
What are the Real-World Applications?
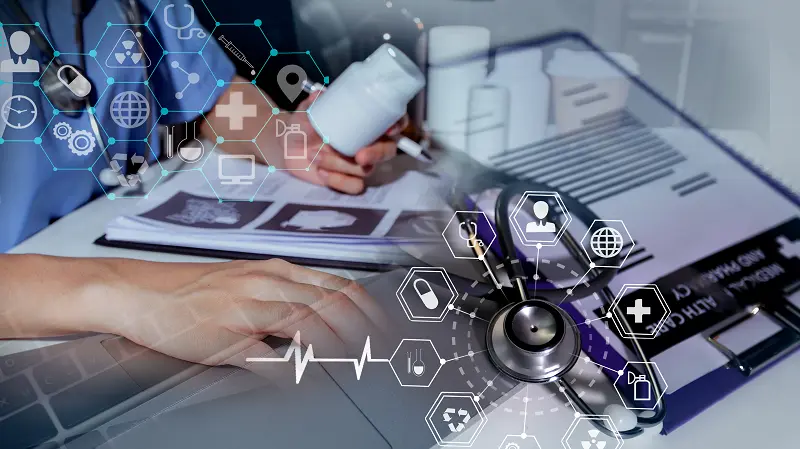
1. Synthetic Data Generation
Generating synthetic data is critical for training AI models without compromising patient privacy. For instance, Generative AI in Healthcare can create synthetic patient records that resemble real-world data while protecting sensitive information.
Example: The Mayo Clinic utilizes synthetic data to train AI models for predictive analytics, ensuring patient confidentiality.
2. Predictive Analytics in Disease Prevention
Generative AI can simulate disease progression scenarios, helping health care providers predict and prevent conditions such as heart disease, diabetes, and cancer. By analyzing patterns, AI can provide early warning signs for interventions.
Example: A generative AI system developed by NVIDIA and King’s College London predicts the growth of brain tumors, improving treatment planning and outcomes.
3. AI-Enhanced Surgeries
Generative AI in healthcare contributes to robotic surgeries by simulating procedures and providing surgeons with detailed, patient-specific 3D models. These simulations enhance precision and reduce the risk of complications.
Statistic: The market for AI in surgical robots is expected to grow from $5.3 billion in 2021 to $14.7 billion by 2028, at a CAGR of 15.1%.
What are the Challenges and Ethical Considerations?
Despite its promise, generative AI in health care faces several challenges:
- Data Privacy and Security: Ensuring patient data is protected remains a top concern. Generative AI systems must comply with regulations such as HIPAA in the U.S. and GDPR in Europe.
- Bias in AI Models: AI models trained on biased data may perpetuate disparities in health care. Addressing this issue requires diverse and representative datasets.
- Regulatory Approval: Deploying generative AI in clinical settings requires rigorous testing and regulatory approval to ensure safety and efficacy.
- Integration with Existing Systems: Integrating generative AI into existing healthcare infrastructure can be complex and resource-intensive.
Future Outlook
The adoption of generative AI in health care is poised to grow significantly in the coming years. With advancements in computational power, data availability, and algorithmic sophistication, generative AI will likely become an integral part of healthcare delivery.
Conclusion
Generative AI in healthcare holds immense potential to address some of the most pressing challenges in health care. From improving diagnostic accuracy to revolutionizing drug discovery, its applications are vast and transformative. However, stakeholders must navigate ethical, regulatory, and technical challenges to fully realize its benefits. As the health care industry continues to embrace AI-driven innovations, generative AI will undoubtedly play a pivotal role in shaping a healthier future.
Ready to embrace generative AI in healthcare? If so, visit Cohort Data and gain insights to drive innovation, efficiency, and better patient outcomes. Explore the possibilities today!