In today’s data-driven world, artificial intelligence (AI) and machine learning (ML) are transforming industries by automating processes, enhancing customer experiences, and optimizing operations. However, for AI models to truly perform at their best, they need high-quality data.
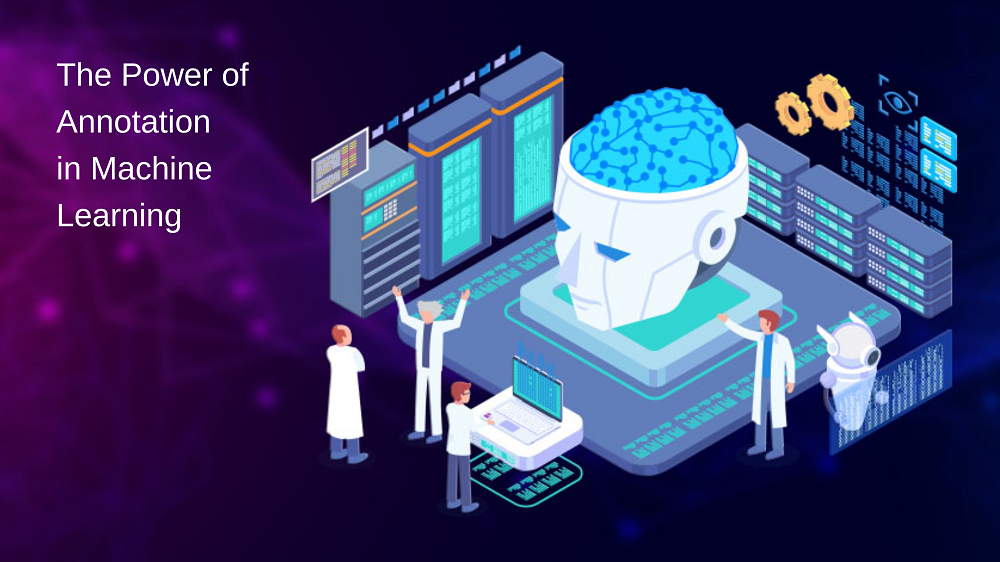
This is where annotation in machine learning becomes essential!
Data annotation and labelling are fundamental steps in building intelligent systems that can understand and interpret data effectively.
What is Data Annotation and Labelling?
At its core, data annotation and labelling involve adding meaningful metadata to raw data. This process is critical in training AI and machine learning models because it enables these models to make sense of the data, they are fed. Whether you are working with images, text, videos, or audio, accurate labelling and annotation allow the AI to recognize patterns and make predictions based on the data.
- Data annotation typically refers to the act of tagging or labelling raw data with relevant information.
- Data labelling focuses on assigning labels to datasets to help machines understand context, patterns, or objects.
For example, in a self-driving car system, data annotation might involve labelling objects like pedestrians, traffic signs, or other vehicles in the camera footage. For a chatbot, it might involve tagging phrases and sentences with appropriate intents and entities to train the system to understand human language.
Why is Data Annotation So Important for Machine Learning?
Data annotation is the bedrock of machine learning. It ensures that AI models learn from high-quality, labelled data, which is crucial for the performance of any model. Without well-labelled data, the model would be unable to make accurate predictions or recognize complex patterns.
In machine learning, supervised learning is one of the most common paradigms, where the model is trained using labelled data. The quality of the training dataset directly impacts the model’s accuracy, and this is where AI data annotation services come into play.
How Do AI Data Annotation Services Work?
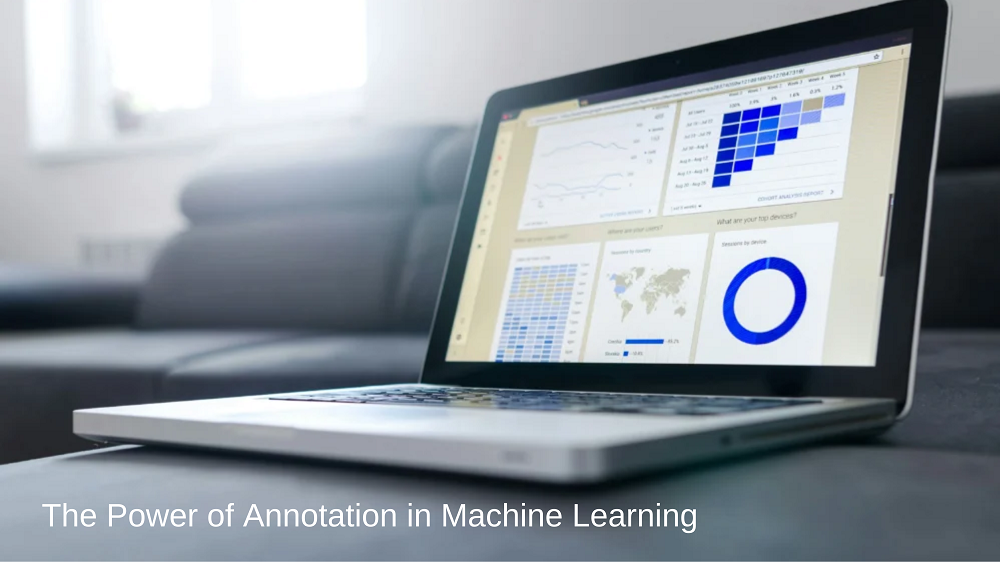
AI data annotation services specialize in labelling large datasets quickly and accurately. These services leverage both human annotators and advanced AI tools to handle the complex task of annotating diverse data types. Here’s how the process typically works:
- Data Collection: The first step is gathering the raw data that needs to be labelled. This could range from text documents, images, audio recordings, and more.
- Annotation Process: Once the data is collected, it is passed to annotation experts who assign the relevant tags, labels, or classifications. Depending on the nature of the data, they may also apply more specific techniques like object detection, segmentation, or sentiment analysis.
- Quality Assurance: After the data has been annotated, it goes through a thorough review to ensure the labels are accurate. This step is crucial in ensuring the quality of the dataset for machine learning.
- Model Training: With the annotated data, the machine learning model can now be trained, tested, and fine-tuned to perform specific tasks such as image recognition, language translation, or customer sentiment analysis.
Types of Data Annotation Services
Different types of AI models require different forms of data annotation. Some of the most common services offered in the field include:
- Image Annotation: Labelling objects in images to train models for computer vision applications like facial recognition or object detection.
- Text Annotation: Labelling text data for natural language processing tasks, such as sentiment analysis, named entity recognition, and part-of-speech tagging.
- Video Annotation: Similar to image annotation, but applied to frames in videos, used in applications such as action recognition or autonomous driving systems.
- Audio Annotation: Transcribing and labelling audio data to improve speech recognition systems or virtual assistants.
- 3D Point Cloud Annotation: Annotating data collected from 3D scanners for applications in robotics and autonomous vehicles.
Benefits of Outsourcing Data Annotation to AI Services
While data annotation is vital for building successful AI applications, it’s also a labour-intensive and time-consuming process. Outsourcing this task to specialized AI data annotation services offers several advantages:
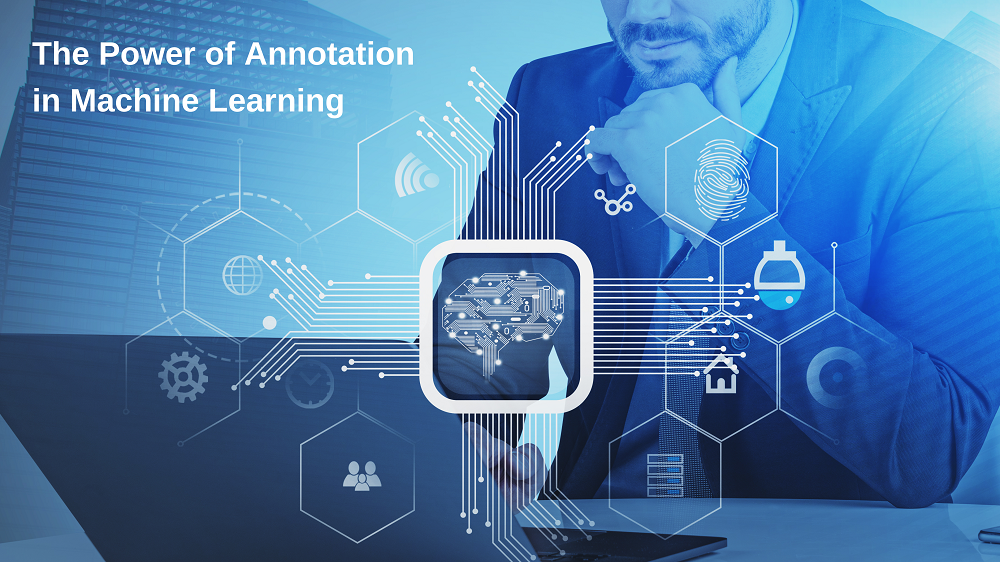
- Speed and Scalability: Annotating vast amounts of data can be time-consuming when done in-house. AI data annotation services, equipped with skilled teams and advanced tools, can scale the process rapidly, meeting tight deadlines and large datasets.
- Quality Control: Professional annotation services ensure the data is labelled accurately, which improves the performance of machine learning models. These services often employ rigorous quality assurance protocols to ensure consistency and accuracy.
- Cost-Effectiveness: By outsourcing data annotation, businesses can save resources and focus on other critical areas of AI development, such as model design and optimization.
Key Takeaways
Whether you’re working on autonomous vehicles, virtual assistants, or customer service chatbots, the right data annotation and labelling can make all the difference in building robust, intelligent systems. So, as AI continues to revolutionize industries, consider leveraging AI data annotation services to ensure that your machine learning models are trained on high-quality, well-annotated data.
At Cohort Data, we specialize in providing accurate, efficient, and scalable data annotation services to help your AI systems reach their full potential. Contact us today to learn more!